'Robotics and AI can have a very positive impact in the world, but we need to make them more reliable, relatable, and sustainable.'
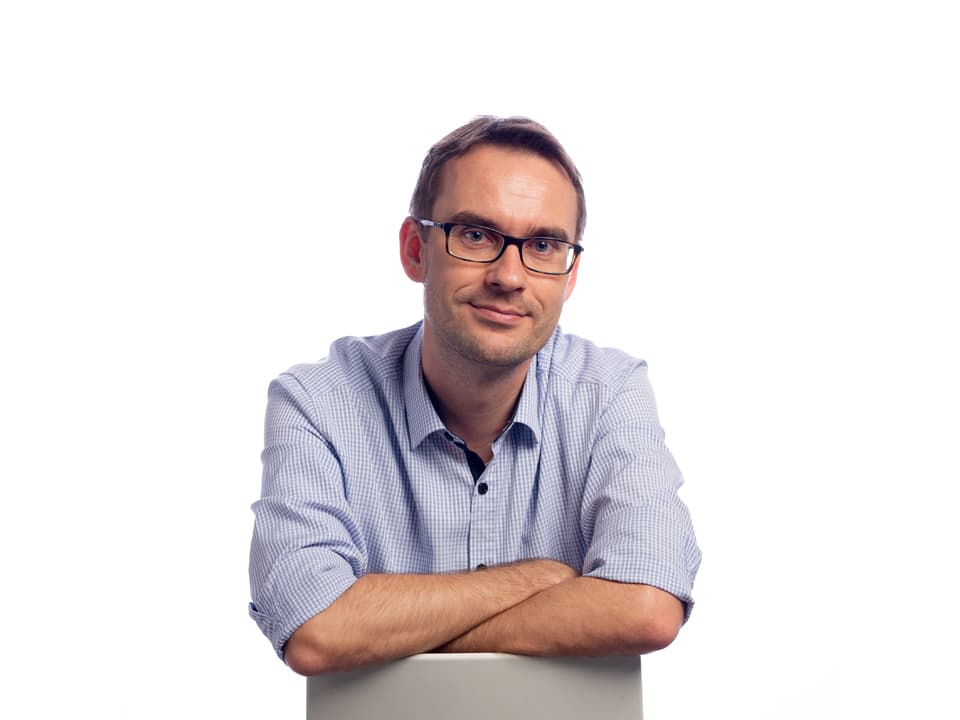
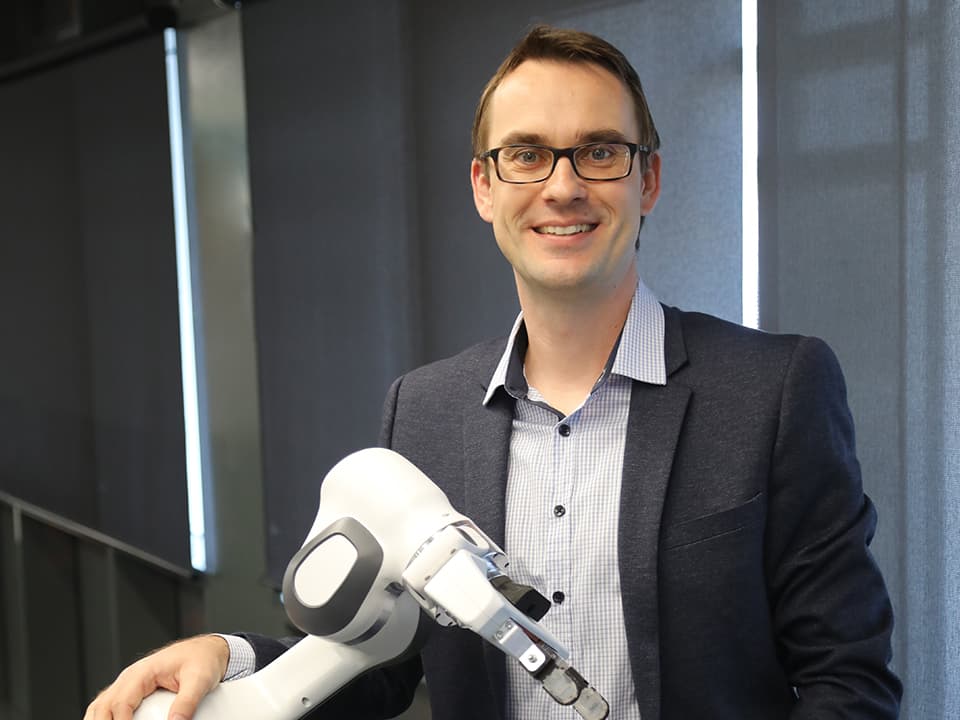
The spark
'I’ve always been a Star Trek fan. The Next Generation series portrayed humans working closely with an android (AI) in a very just society where technology usually played a highly positive and enabling role. I think that got me motivated to help create such a society in reality. As a result, in my early teenage years I was fascinated by computer programming.'
Research aim
'My research goal is to create reliable, relatable, and sustainable robotics and AI that can understand and interact skilfully with the surrounding world and work closely with humans to improve our lives.'
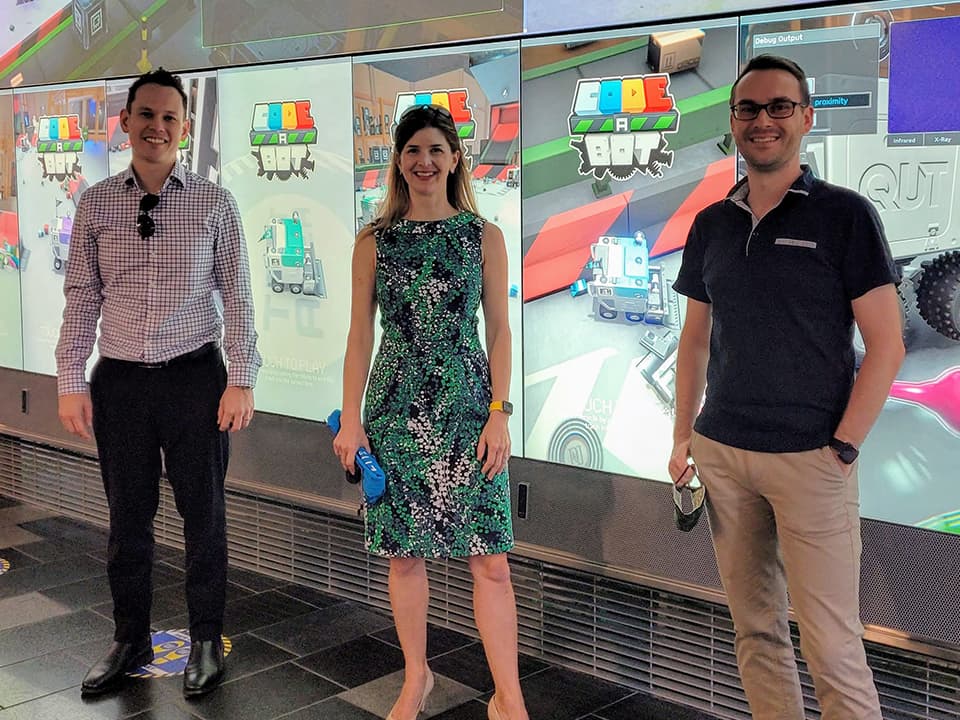
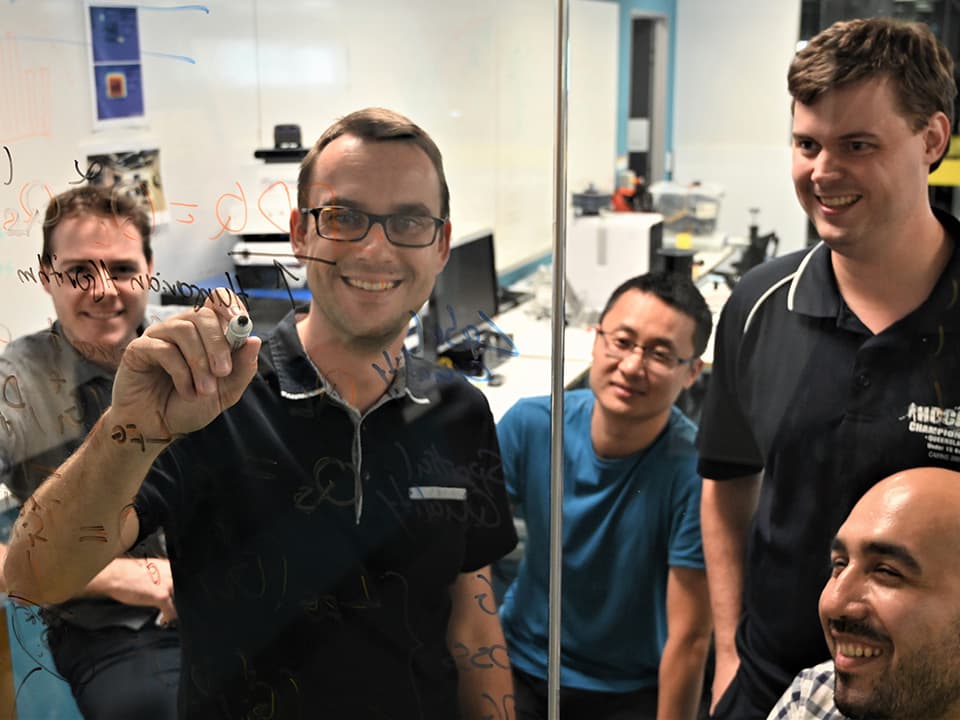
Real-world implications
'Robotics and AI technology can help solve humanity’s biggest demographic, societal, environmental and economic challenges like those related to an ageing society, climate change, and food security.'
The challenge
'Collaborating with researchers from neuroscience, psychology, law, design, philosophy and other disciplines should help computer scientists and engineers develop smarter robots, address pressing issues like fairness and bias in AI to ensure all parts of our society benefit equitably from new technologies, while preventing unintended negative impacts.'
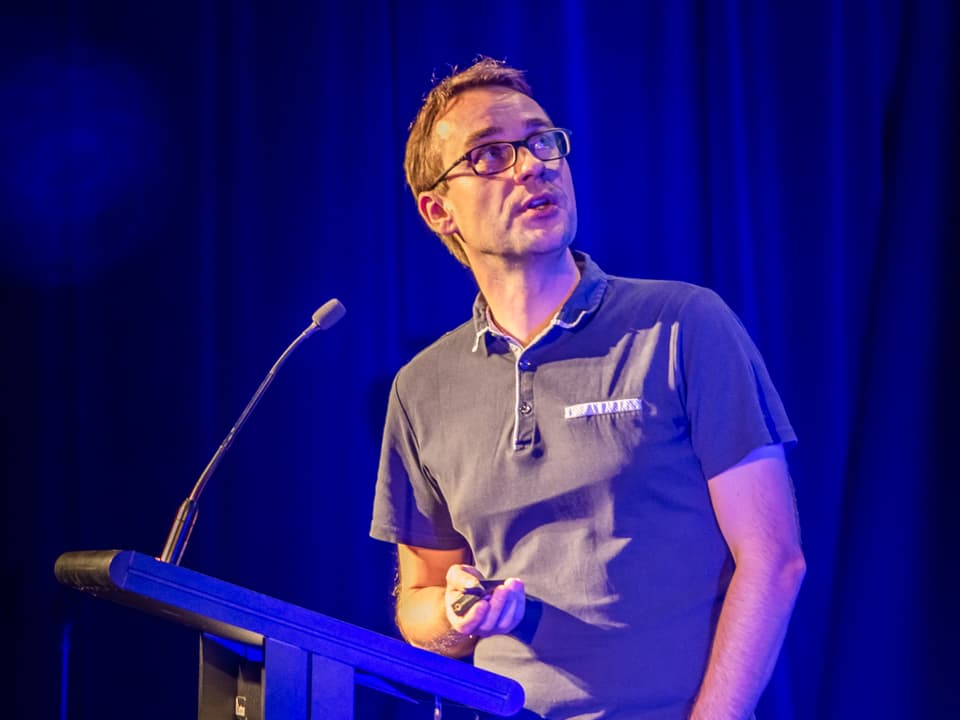
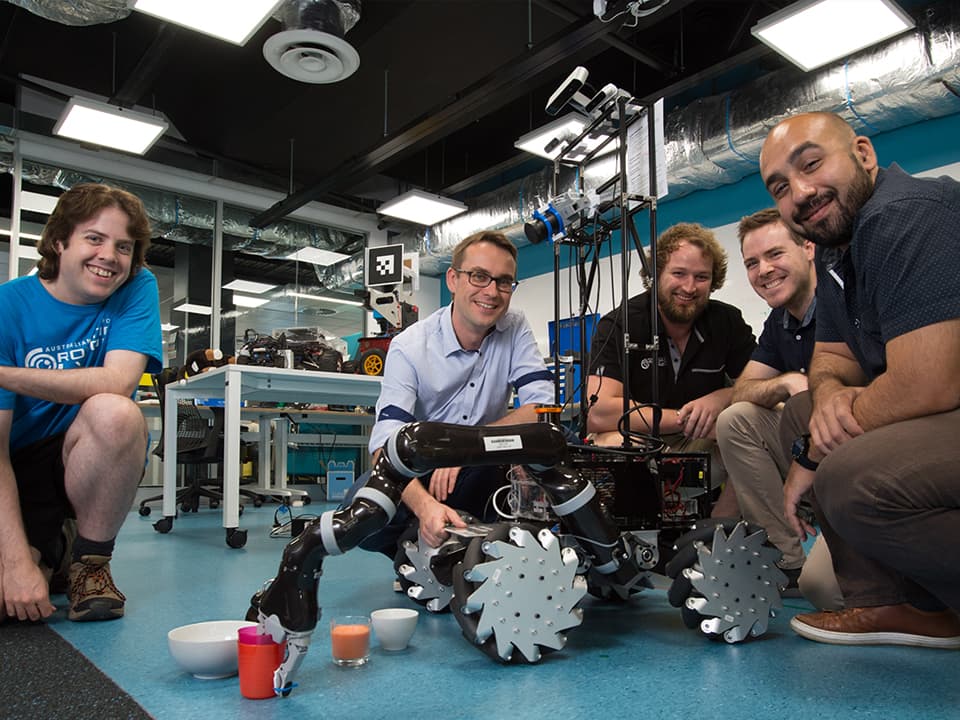
Teaching
'I love seeing students having lightbulb moments when they understand something for the first time. It is very fulfilling to guide them along their career pathway, whether they are starting as an undergraduate or advancing through research or industry. It is always a stimulating experience to discuss new ideas with students and to learn from each other.'
Key collaborators
Engineers and computer scientists are great at advancing technologies, but we are not well trained to predict its impact at small and large scales. We need to do this in collaboration with other discipline experts.
The exchange of ideas, expertise, and viewpoints is what makes collaborative research so interesting. Being at the forefront of research, contributing new knowledge and continuously learning what other researchers have discovered is extremely exciting. In my current work, I collaborate with colleagues from the University of Adelaide, DeepMind, and Amazon, among many others.
Key Achievements
- My pioneering research into robust methods for graph-based simultaneous localisation and mapping (SLAM) and the accompanying open-source implementation were integrated into the real-time appearance-based mapping (RTAB-Map) package of the Robot Operating System (ROS)—a huge ecosystem used for anything related to robotics, including warehouse and mobile robots.
- My co-authors and I were the first to demonstrate the efficacy of deep learned features for place recognition despite severe changes in appearance and viewpoint.
- My research in semantic scene understanding and object-based semantic SLAM cumulated in the recent development of a first-of-its-kind object-based semantic SLAM system. This research should help robots understand which objects are in their environment, the position of the objects, and their relations. Ultimately, I hope this enables competent domestic service robots that can handle many objects and help us with everyday tasks.
Key publications
Suenderhauf, Niko, Brock, Oliver, Scheirer, Walter, Hadsell, Raia, Fox, Dieter, Leitner, Jurgen, et al. (2018) The limits and potentials of deep learning for robotics. International Journal of Robotics Research, 37(4 - 5), pp. 405-420.
Nicholson, Lachlan, Milford, Michael, & Suenderhauf, Niko (2019) QuadricSLAM: Dual quadrics from object detections as landmarks in object-oriented SLAM. IEEE Robotics and Automation Letters, 4(1), Article number: 8440105 1-8.
Suenderhauf, Niko, Shirazi, Sareh, Dayoub, Feras, Upcroft, Ben, & Milford, Michael (2015) On the performance of ConvNet features for place recognition. In Burgard, W (Ed.) Proceedings of the 2015 IEEE/RSJ International Conference on Intelligent Robots and Systems (IROS 2015). IEEE, United States of America, pp. 4297-4304.
Centre for Robotics
The QUT Centre for Robotics builds on a decade of investment in robotic research and translation, which has been funded by QUT, Australian Research Council, Queensland Government, cooperative research centres and industry.